Anticipating Cryptocurrency Prices with Machine Learning
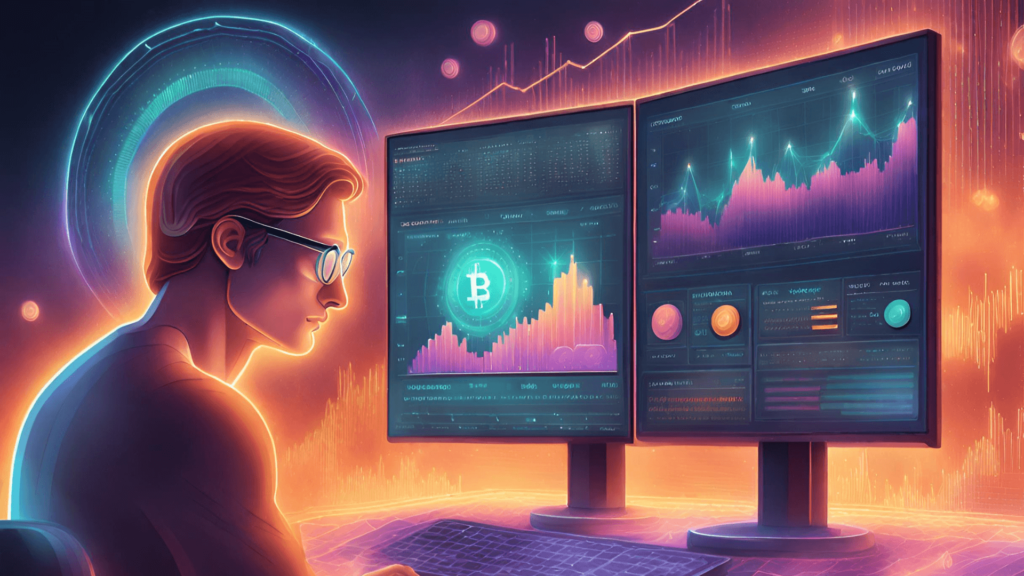
Understanding the Emerging World of Cryptocurrencies
Predicting the Future: Anticipating Cryptocurrency Prices with Machine Learning | Cryptocurrencies, such as Bitcoin and Ethereum, are digital assets that use cryptography for secure transactions. These digital currencies are not controlled by any central authority, making them resistant to government interference and censorship. The growing popularity of cryptocurrencies can be attributed to their potential to revolutionize various industries, including finance, technology, and even governance.
The need to predict cryptocurrency prices
Given the highly volatile nature of cryptocurrency markets, investors are constantly looking for ways to accurately predict price movements. By anticipating these price trends, they can make informed decisions and maximize their returns. Cryptocurrency price prediction has become crucial for both short-term traders and long-term investors looking to optimize their portfolios. Machine learning presents a promising approach to address this challenge.
Overview of machine learning and its potential in forecasting
Machine learning is a branch of artificial intelligence that allows computers to learn and make predictions or decisions without being explicitly programmed. It involves developing algorithms that can analyze and interpret large data sets to identify patterns and make predictions based on historical data. When applied to cryptocurrency price prediction, machine learning algorithms can detect trends and patterns that may be invisible to the human eye, allowing for more accurate forecasts.
Fundamental Factors Affecting Cryptocurrency Prices

Market capitalization and volume
Exploring the impact of market capitalization on price trends
Market capitalization refers to the total value of a cryptocurrency. It takes into account the total supply of coins circulating in the market and their current price. Cryptocurrencies with larger market caps tend to be more stable and less prone to drastic price fluctuations. Analyzing the impact of market capitalization on price trends can provide valuable information on future price movements.
Analysis of volume as an indicator of price movements
Volume represents the number of stocks or currencies traded within a specific time period. High trading volumes usually indicate increased investor interest and can lead to significant price movements. Analyzing volume patterns can help predict short-term price fluctuations and identify potential trends in the market.
Understand the interaction between market capitalization and volume
The interaction between market capitalization and volume is essential for predicting cryptocurrency prices. While large volume can drive short-term price movements, sustained changes in market capitalization can have a long-term impact on prices. Examining the relationship between these two factors can provide valuable information about future price trends.
Economic factors and global market trends
Examining the macroeconomic variables that influence cryptocurrency prices
Cryptocurrency prices are influenced by several macroeconomic variables, including interest rates, inflation, and GDP growth. These variables can affect investor sentiment and cryptocurrency market demand. By examining these economic factors, machine learning models can identify patterns and correlations that help forecast future price movements.
Revealing the relationship between cryptocurrencies and traditional markets
Cryptocurrencies have become increasingly interconnected with traditional financial markets. Factors such as stock market performance, geopolitical events, and global economic trends can affect cryptocurrency prices. Understanding these interrelationships is crucial to accurately predict cryptocurrency prices using machine learning algorithms.
The role of government policies and regulations in price predictions
Government policies and regulations, both favorable and unfavorable, have a significant impact on cryptocurrency prices. Positive regulatory developments, such as the legalization of cryptocurrencies, can drive prices up, while negative regulatory actions can lead to sharp price declines. Incorporating government policies and regulations into machine learning models can improve the accuracy of price predictions.
Technological advances and adoption
Assessing the Influence of Technological Advances on Cryptocurrency Prices Technological advances, such as the implementation of blockchain technology or the introduction of cryptocurrencies, can have a profound impact on cryptocurrency prices. Machine learning algorithms can analyze the impact of these technological developments on price trends and identify opportunities for price prediction.
Analyzing cryptocurrency adoption patterns and their impact on prices
The adoption of cryptocurrencies by businesses and individuals plays a crucial role in determining their market value. Higher adoption rates indicate greater demand and can drive up prices. Machine learning models can analyze adoption patterns and predict future price movements based on the rate of cryptocurrency adoption.
Identify the importance of network effects in price prediction
Network effects refer to the phenomenon in which the value of a cryptocurrency increases as the number of users and participants in its network grows. Machine learning algorithms can analyze network effects and predict the impact of user growth on cryptocurrency prices, providing valuable information for price forecasting.
Machine Learning Models for Cryptocurrency Price Prediction

Time Series Analysis Approaches
Taking advantage of autoregressive integrated moving average (ARIMA) models
ARIMA models are widely used in time series analysis to forecast future values based on past observations. These models capture trends and seasonality in the data, making them suitable for predicting cryptocurrency prices. By leveraging historical price data, ARIMA models can provide accurate short-term price predictions.
Exploring the application of autoregressive conditional heteroskedasticity (ARCH) models
ARCH models are specifically designed to capture volatility clustering in time series data. Given the high volatility of cryptocurrencies, ARCH models can be effective in predicting short-term price volatility. By incorporating ARCH models into machine learning algorithms, more accurate price predictions can be obtained.
Combining time series models to improve accuracy
By combining different time series models, such as ARIMA and ARCH, machine learning algorithms can leverage the strengths of each model and improve the accuracy of price predictions. Ensemble methods, such as averaging or stacking, can be used to obtain more reliable forecasts.
Sentiment analysis techniques
Using Natural Language Processing (NLP) for Sentiment Analysis
Natural language processing (NLP) techniques can analyze textual data, such as social media posts or news articles, to determine opinions and extract valuable information. By analyzing sentiment from cryptocurrency-related discussions and news, machine learning models can predict short-term price movements based on investor sentiment.
Leveraging social media data to predict price movements
Social media platforms, such as Twitter and Reddit, are rich sources of information and sentiment regarding cryptocurrencies. Machine learning algorithms can analyze social media data in real time to predict and quantify the impact of social media discussions on price movements. By integrating social media data into predictive models, more accurate price forecasts can be achieved.
Integration of sentiment analysis with machine learning models
By integrating sentiment analysis with machine learning models, such as regression or neural networks, the impact of sentiment on cryptocurrency prices can be quantified. Sentiment analysis provides a valuable additional dimension to predicting price movements and can significantly improve the accuracy of machine learning models.
Machine learning algorithms for price forecasting
Overview of Regression Models and Their Application in Price Prediction
Regression models, such as linear regression or polynomial regression, can be used to predict cryptocurrency prices based on historical data and relevant predictors. These models identify relationships and correlations between variables to generate price forecasts. By training these models on large data sets, machine learning algorithms can accurately predict short- and long-term price movements.
Unsupervised Learning Techniques to Identify Cryptocurrency Patterns
Unsupervised learning techniques, such as clustering or dimensionality reduction, can identify underlying patterns and structures in cryptocurrency price data. By grouping similar price patterns together, machine learning algorithms can make predictions based on historical trading patterns and identify potential trends or anomalies.
Set of methods to improve accuracy and make robust predictions
Ensemble methods involve combining multiple machine learning models to obtain more accurate and robust predictions. Techniques such as bagging, boosting, or stacking can be used to take advantage of the diversity of multiple models and improve the accuracy of cryptocurrency price forecasts. By combining different algorithms, a more complete and reliable prediction can be achieved.
Challenges and Limitations in Cryptocurrency Price Prediction
Volatility and non-linear price movements
Understanding the Unique Volatility Dynamics of Cryptocurrencies
Cryptocurrencies are known for their high volatility, with prices fluctuating significantly over short periods. This volatility poses a challenge to accurate price prediction, as traditional models may not capture non-linear price patterns. Advanced techniques, such as neural networks or deep learning models, can be employed to address the unique volatility dynamics of cryptocurrencies.
Handling non-linear price patterns using advanced techniques
Non-linear price patterns in cryptocurrencies require sophisticated modeling techniques that can capture complex relationships. Machine learning algorithms, such as recurrent neural networks or short-term memory networks, can capture non-linear dependencies and make more accurate price predictions by learning from historical price data.
Mitigate risk and uncertainty in price predictions
Despite advances in machine learning and predictive analytics, price predictions in cryptocurrency markets always carry a certain degree of risk and uncertainty. Investors and traders should be aware of the limitations of predictive models and employ risk management strategies to mitigate potential losses. Diversification, stop-loss orders, and thorough fundamental analysis should complement predictive models to make informed investment decisions.
Data quality and availability
Address issues of incomplete and inaccurate data
Cryptocurrency price data may suffer from incompleteness or inaccuracies due to the decentralized nature of these markets. It is critical to address these data quality issues before training machine learning models for price prediction. Data preprocessing and cleaning techniques, such as outlier removal and missing data imputation, are essential to ensure accurate and reliable predictions.
Exploring data sources for robust price prediction models
Choosing the right data sources is crucial to creating robust cryptocurrency price prediction models. Historical price data from reputable exchanges, combined with additional relevant data such as trading volume or sentiment indicators, can improve the accuracy of machine learning algorithms. It is important to consider the quality, reliability and integrity of the selected data sources.
The importance of data preprocessing and feature engineering
Data preprocessing and feature engineering play a vital role in developing accurate machine learning models for price prediction. Techniques such as normalization, scaling, or feature extraction can improve the performance of predictive models. Feature engineering involves selecting and transforming relevant predictors to improve the predictive power of the model.
Ethical and regulatory considerations
Ensure the responsible use of predictive models in crypto markets
When leveraging machine learning for cryptocurrency price prediction, it is essential to ensure the responsible and ethical use of predictive models. Transparency, fairness and accountability should be guiding principles in the development and implementation of predictive models in crypto markets. Machine learning algorithms should be used to aid decision making rather than completely replacing human judgment.
Privacy concerns and potential risks of manipulation in data-driven predictions
The use of personal data in machine learning models raises privacy concerns, especially in the context of crypto markets. Safeguarding user privacy and protecting data from potential misuse should be a priority. Additionally, data-driven predictions can be vulnerable to risks of manipulation, such as fake news or coordinated trading strategies. Regulatory frameworks and strict data protection measures can help mitigate these risks.
Compliance with regulatory frameworks for reliable predictive analysis
The regulatory landscape surrounding cryptocurrencies is continually evolving. Adhering to regulatory frameworks and complying with relevant laws and guidelines is crucial to conducting reliable predictive analysis in the crypto markets. Compliance with anti-money laundering (AML) regulations, know-your-customer (KYC) procedures, and security requirements is essential for reliable predictive models.
Summary and Future Perspectives
Recapping key findings in cryptocurrency price prediction
In this article, we explore the emerging world of cryptocurrencies and the need to predict their prices. We discuss the fundamental factors that affect cryptocurrency prices, such as market capitalization, volume, economic variables, and technological advancements. We also explore machine learning models for cryptocurrency price prediction, including time series analysis, sentiment analysis, and regression algorithms. We highlight challenges and limitations in price prediction, such as volatility, data quality, and ethical considerations.
Reflecting on the potential impact of machine learning on crypto markets
Machine learning has the potential to revolutionize cryptocurrency markets by providing accurate and timely price predictions. By leveraging large amounts of real-time and historical data, machine learning algorithms can uncover hidden patterns and trends, allowing investors and traders to make informed decisions.
Future Directions and Evolving Trends in Cryptocurrency Price Forecast
As technology continues to advance and new developments emerge in the world of cryptocurrencies, the field of price prediction will evolve even further. Advances in deep learning, natural language processing, and decentralized finance (DeFi) will shape the future of cryptocurrency price forecasting. Adapting machine learning models to incorporate these emerging trends will be crucial to staying ahead in the fast-paced world of crypto markets.
Frequently asked questions (FAQ)
1. Can machine learning accurately predict cryptocurrency prices in the long term?
*Machine learning can provide valuable insights into cryptocurrency price trends, but the accuracy of long-term predictions depends on several factors, including market dynamics, technological advances, and regulatory changes. It is important to consider predictive models as a tool in a comprehensive investment strategy.
2. What are the main challenges we face in accurately predicting cryptocurrency prices?
* Accurately predicting cryptocurrency prices faces challenges such as high volatility, non-linear price movements, data quality issues, and ethical considerations. These challenges require sophisticated modeling techniques, robust data sources, and compliance with regulatory frameworks for reliable predictions.
3. How can investors practically incorporate machine learning predictions into their trading strategies?
* Investors can incorporate machine learning predictions into their trading strategies by using them as an additional source of information. It is important to combine predictive models with fundamental and technical analysis, risk management strategies and human judgment. Machine learning predictions can complement investment decisions, but should not replace thorough analysis and due diligence.
4. What are the ethical considerations when using machine learning for cryptocurrency price prediction?
*Ethical considerations include ensuring responsible use of predictive models, safeguarding user privacy, and complying with regulatory frameworks. Transparency, fairness, and accountability should guide the development and deployment of machine learning models in crypto markets.
5. Which machine learning approach is most effective for forecasting cryptocurrency prices?
*The effectiveness of machine learning approaches for cryptocurrency price forecasting depends on several factors, including the specific prediction task, data availability, and the interaction of different factors affecting cryptocurrency prices. No single approach is universally superior, and a combination of time series analysis, sentiment analysis, and regression algorithms can produce the most accurate predictions.
Predicting the Future: Anticipating Cryptocurrency Prices with Machine Learning